A more parameter-efficient bottleneck for EfficientNet!
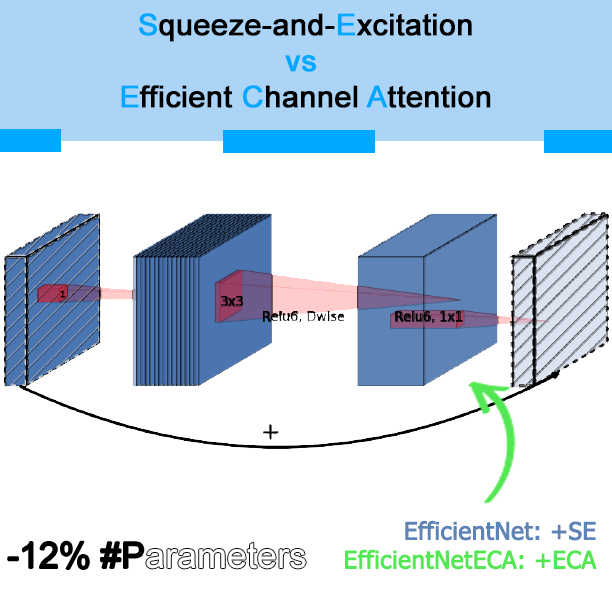
Introduction
CNN are great blablabla… Let’s get to the point. SOTA for image classification on Imagenet is EfficientNet with 88.5% top 1 accuracy in 2020. EfficientNet comes from MobileNet V2. In this article, I introduce a combination of EfficientNet and Efficient Channel Attention (ECA) to highlight the results of the ECA paper from Tianjin/Dalian/Harbin universities.
Linear Bottleneck
EfficientNet is based on MobileNetV2. MobileNetV2 is composed of multiple blocks which are called linear bottlenecks or inverted residuals (they’re almost the same). More informations here, or in the mobilenetv2 paper.
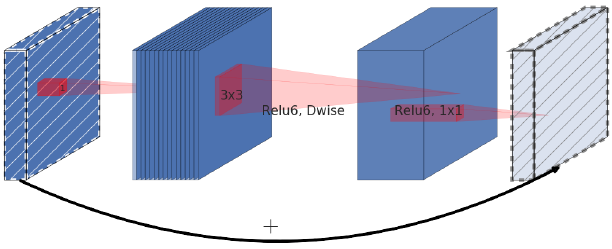
Linear Bottleneck is a residual layer composed of one 1x1 convolution, followed by a 3x3 depthwise convolution, then finally a 1x1 convolution. It is used as a basis for EfficientNet because of its efficiency (yeah). 1x1 convolutions don’t require much parameters so do 3x3 depthwise convolutions, and they’re fast to compute.
However, linear bottlenecks don’t have an attention layer.
EfficientNet: Linear Bottleneck + Squeeze Excitation
EfficientNet solves this problem by adding an attention layer. In 2018~2019, the trending attention layer for convolutions is Squeeze Excitation (SE). The same layer is used in MobileNetV3.

So it’s almost the same, 1x1conv, 3x3dwise, SE, 1x1conv, this is what powers SOTA.
SE: Provided a C channels feature map, SE produces C scalars to be multiplied with each channel. You have a feature map of shape B*C*H*W, you apply average pooling: you get B*C*1*1, then a 1x1 convolution (same as a simple linear layer multiplying with weights and summing everything with biases), an activation layer, another 1x1 and a sigmoid. You then get B*C*1*1 positive scalars to be multiplied with your B*C*H*W feature map, which effectively produces an attention over some channels of your feature map.
Problem is, SE is highly parametric. It undoubtedly enhances the accuracy but multiplying everything with everything isn’t necessary “efficient”. EfficientNetB0 has 5.3M #Params and 0.39B #FLOPS.
We reproduced Efficient Net B0 architecture with some tweaks on the hyperparameters (optimizer, augmentation..) for our usage (fast non-dataset specific learning). EfficientNet results are hard to reproduce on low/middle-end hardware (it’s also reported here). Working re-implementations of training in Pytorch include rwightman and kakaobrain’s fast-autoaugment. This is our result:
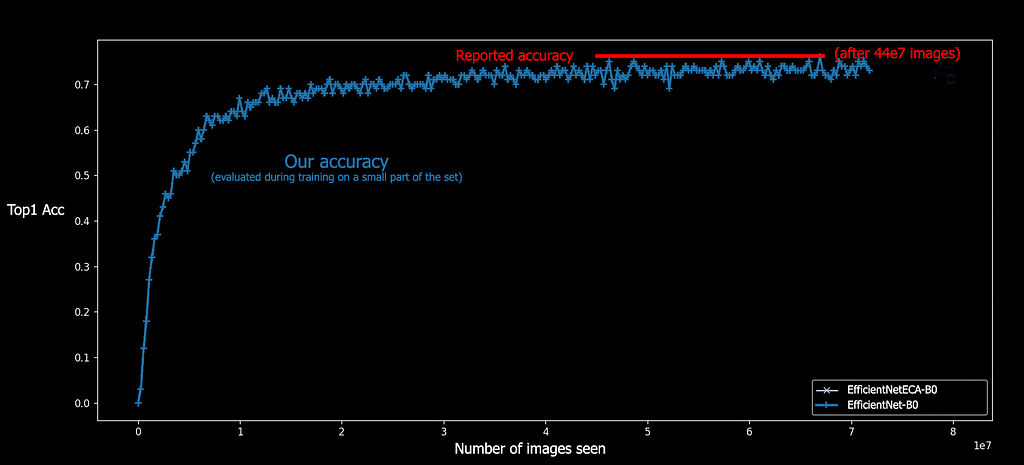
As you can see, we didn’t manage to reproduce the reported accuracy with our setup, but it doesn’t really matter for this experiment.
EfficientNetECA: Linear Bottleneck + Efficient Channel Attention
ECA is an attention layer from 2019~2020. [The paper]. It’s an extremely simple attention layer which performs pretty well as you can see:

ECA models are more parameter-efficient than SENet-50 (SEResNeXt-50) which added +10% parameters compared to ResNet-50, while ECANet-50 adds less than 1% in parameters and GFLOPS (though ECANet does reduce the FPS by 3%, while SENet reduced the FPS by 13%). This inefficiency was already reported in the SE paper.
It’s basic, ECA captured what they called “local cross-channel interaction”. Which can be translated to “Conv1D with low kernel size”.
ECA: Provided a C channels feature map, ECA produces C scalars to be multiplied with each channel. You have a feature map of shape B*C*H*W, you apply average pooling: you get B*C*1*1, then a 1D-convolution with a low kernel_size (3 for example), no bias, sigmoid, and voilà. You then get B*C*1*1 positive scalars to be multiplied with your B*C*H*W feature map, which effectively produces an attention over some channels of your feature map.
And that’s it. Now introducing: Linear Bottleneck ECA. What is it? It’s Linear Bottleneck SE (EfficientNet main block), with an ECA layer in place of the SE layer. How it performs?
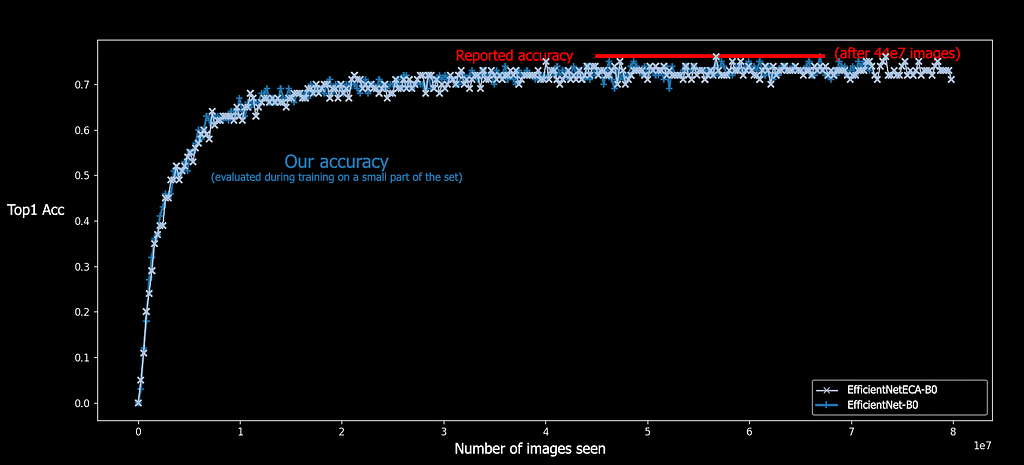
Ok, it’s the same, what’s the point? Well B0 has 5,288,548 parameters. ECA-B0 has 4,652,084 parameters. We reduced the number of parameters of the SOTA architecture by 12%!
After 66.560.000 images seen (52 epochs), for one training, on our setup, B0 without any attention layers is at 72.57%, B0-SE is at 73.17% and B0-ECA is at 73.31%. This is evaluated on the whole Imagenet validation set. It’s not significant but it supports the hypothesis that ECA performs better or the same than SE, with much less parameters.
Tl;dr
You don’t use channel attention? Pffft, cringe! Use it. You use SE? Try ECA: less parameters, more efficiency. We trained EfficientNetB0 with ECA layer instead of SE and it performed similarly with 12% less parameters.
Conclusion / Further works
ECA layer seems to be a great way to improve all models which don’t use an attention layer, or which use SE in terms of parameter-efficiency.
We don’t have the hardware to train EfficientNet up to it’s reported accuracy but we hope someone will try training a NoisyStudent-FixEfficientNetECA-L2. We’ll do it by ourselves if we manage to find the gpu-day for this usage, or we wish that the ECA team will try it (they already published the results and their github for ECA_MobileNetV2), stay tuned!
_____________________________________
More plots
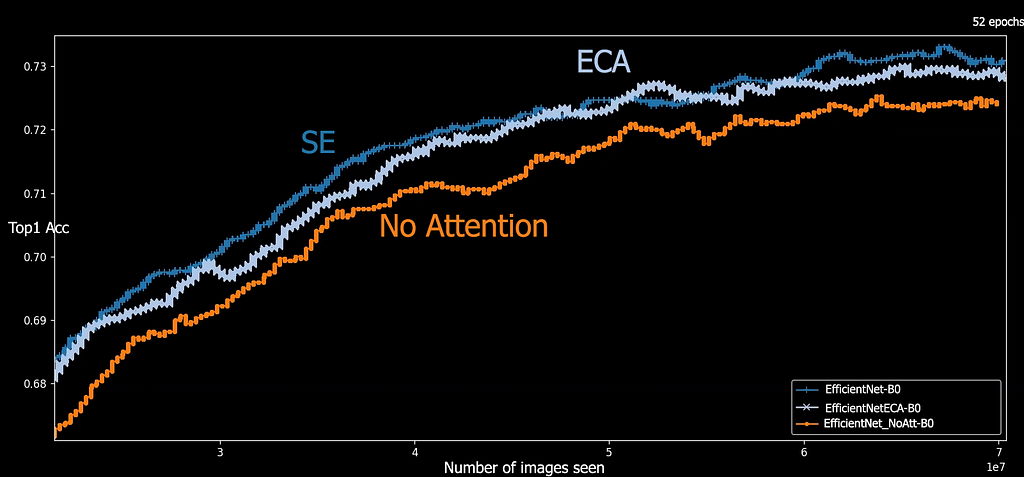
We can see that SE seems to perform better at the beginning of training (in terms of iteration), even though a complete evaluation on the whole set after 52 epochs puts ECA ahead.
___
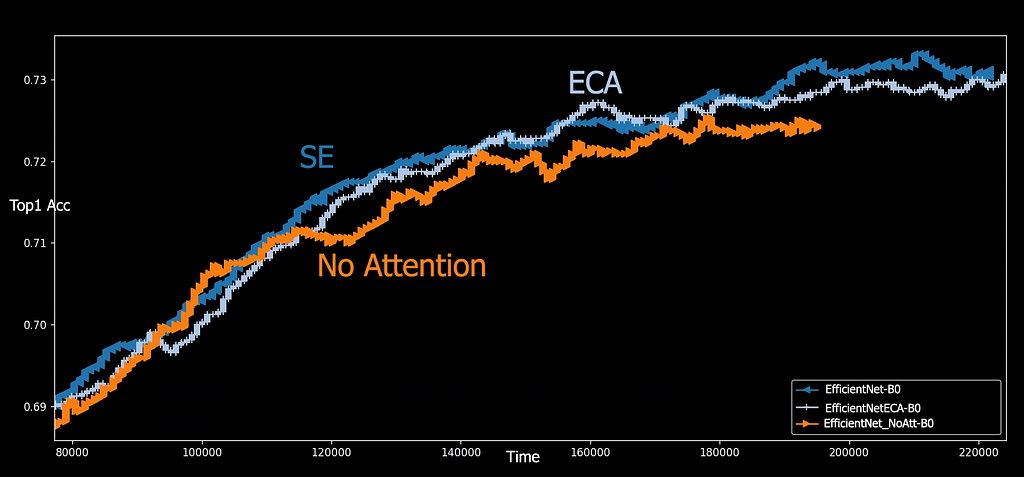
In terms of training speed there’s no clear winner, it looks like SE performs better at the end of the training.
Addendums
Addendum 1 (2020/07/22): YoloV4 (SOTA or almost in early 2020) uses CBAM/SAM, which according to the ECA paper is worst than ECA. It looks like using ECA instead of SAM could improve the current SOTA in both image classification and object detection. ECA paper showed that ECA improves RetinaNet and Faster R-CNN.
Addendum 2 (2020/07/24): Of course accuracies would need to be evaluated more properly, ideally by averaging it on multiple trainings on a completely working reproduction of the SOTA. This article is just a quick ablation study, it’s not as rigourous as research papers.
Addendum 3 (2020/07/25): It’s worth noting that attention layers won’t improve your network massively. SE improved Resnet50 accuracy by 1.51% (in absolute value) and ECA improved it by 2.28%. It’s an easy improvement but on a custom dataset with a custom network you’ll probably see more improvements by modifying other hyperparameters.
Addendum 4 (2020/07/28): +plots, +code
Addendum 5 (2020/10/12): Other results could indicate that ECA, while being more parameter-efficient and still better than no attention, could have a lower score than SE on some trainings.
Code
- ECA: BangguWu github
- SE Linear Bottleneck: lukmelas, rwightman, fast-autoaugment
____________________________________________
This article was written on Medium, by Elie D, for https://www.hyugen.com/en. We are working on AGI.
Support us 💙
You can follow us on Twitter to show support and to see all news (no tweet-spam, only news), or here on Medium.
Date: 2020/07/21